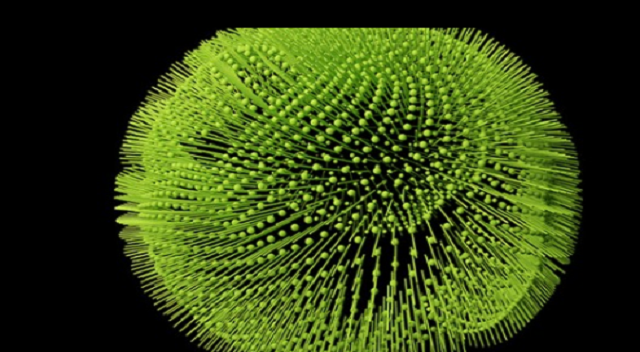
Deloitte
Deloitte
Services
Cognitive Advantage
Driving real business outcomes with cognitive technology solutions
We’ve anticipated it for years, and now it’s becoming reality: Emerging technologies are finally able to emulate and augment the power of the human brain. That has big implications for the business world, which is still coming to terms with the opportunities presented by the enormous amount of data pulsing through markets, individual businesses, and more. Conventional technology is not up to the task of extracting the full value of this mountain of data. But the promise of cognitive technology solutions is clear.
Why now?
The dawn of the cognitive era creates newer, and in many cases bigger, opportunities for businesses to generate value from their data, in tandem with analytics advances. Imagine helping your workers take an “amplified intelligence” approach that combines their own insights with machine-generated insights to focus on what matters most: Improving core operations, building new assets, and enabling better outcomes for your organization and its customers. That’s the cognitive advantage. And with big advances in cognitive capabilities, it’s finally within reach.
How we can help
Our Cognitive Advantage offerings are designed to help organizations transform decision-making, work, and interactions through the use of insights, automation, and engagement capabilities. Our offerings are tailored to be industry-specific and powered by our cognitive platform.
Perspectives
Artificial intelligence (AI) for the real world
A new report in Harvard Business Review
From answering everyday customer queries to finding medical cures and breakthrough treatments, cognitive technologies are helping to solve today’s toughest business problems. Where—and how—are companies having the most success? In this Harvard Business Review article, Tom Davenport and Rajeev Ronanki present their insights from Deloitte’s study of 152 real-world cognitive projects and discuss how it’s wiser to take incremental steps with the currently available technology while planning for transformational change in the not-too-distant future.
Cognitive technologies in action
The promise of AI and other cognitive technologies is enticing companies to take on aggressive new initiatives. So what’s the secret to achieving the best results? Authors Davenport and Ronanki share how to apply cognitive technologies from robotics to deep learning in bold new ways, based on their study of 152 cognitive projects and the results of Deloitte’s 2017 state of cognitive survey.
Taking an incremental approach—rather than a transformative approach—helps organizations avoid potential setbacks. In fact, “low-hanging fruit” projects that streamline business processes and augment, rather than replace, human capabilities are much more likely to be successful than the most highly ambitious projects. Here, you’ll see how organizations are improving products and creating new ones, making better decisions, and freeing up workers to be more creative using cognitive technologies.
Laying the foundation
As companies begin to the test the waters and experiment with cognitive tools, they face significant obstacles in development and implementation. In this article, the authors outline how forward-thinking organizations can achieve their objectives by following a four-step process:
- Understanding the technologies: Learn what technologies can help you achieve desired results; investing in the right capabilities is critical to achieving successful outcomes.
- Creating a portfolio of projects: e v a luate your organization’s needs and develop a portfolio of projects in areas that can benefit most from cognitive technologies.
- Launching pilots: Create pilot projects for cognitive applications before introducing throughout the enterprise.
- Scaling up: Facilitate collaboration between technology experts and business process owners to take cognitive to the next level.
Download the article to find out more about laying the foundation for AI success and to learn from real-world examples.
Cognitive technologies survey
What else can you learn from leaders who are excited about AI and cognitive technologies? Find out what’s working and what’s not in the 2017 Deloitte State of Cognitive Survey. You’ll see where organizations are making the most significant investments and look into the business processes undergoing transformative change.
Cognitive advantage: smarter insights, stronger outcomes
Cognitive technologies allow organizations to reimagine how humans and machines work together, explore new possibilities, and overcome business challenges. Realizing value comes from a combination of complimentary initiatives: mastering technology, identifying the right data, bringing science to the business issue, and updating how work gets done. At Deloitte, we’re helping our clients develop a “Cognitive Advantage” through a design thinking process that drives smarter insights and stronger outcomes.
Robotics & Cognitive Automation
Enable machines to augment human actions and judgement with robotics and cognitive technologies.
What the advantage looks like: Perform tasks
Transformative change: Automate repeatable tasks to improve efficiency
Flexibility: Unchain profits from scale constraints to increase enterprise flexibility
New competencies: Engage existing talent to focus on higher-value tasks
Cognitive Insights
Identify opportunities for growth, diversification, and efficiencies by creating large-scale organizational intelligence with pattern detection and the ability to analyze multiple data sources.
What the advantage looks like: Make decisions
New growth: Uncover hidden patterns to identify new opportunities for innovation
Evidence-based decision: Apply a science-based decision-making process informed by deeper insights
Timely action: Push real-time, contextual insights to decision makers at relevant moments
Cognitive Engagement
Use intelligent agents and avatars to deliver mass consumer personalization at scale and smarter, more relevant insights to amplify end-user experience.
What the advantage looks like: Take action
Optimized consumer behavior: Drive consumer behavior by delivering hyper-personalization at scale
Next-gen customer experience: Deploy personalized digital assistants to interact with customers
Ubiquitous engagement: Generate personalized and contextual recommendations to consumers
Big ideas
Read some of most recent thought leadership to learn more about how cognitive technology solutions and analytics are transforming business.
- The rise of cognitive work (re)design: Applying cognitive tools to knowledge-based work
- Time to move: From interest to adoption of cognitive technology
- How automation and cognitive technologies increase government efficiencies
How automation and cognitive technologies increase government efficiencies
- Beyond “doing something cognitive”: A systematic approach to implementing cognitive technologies
A systematic approach to implementing cognitive technologies
A systematic approach to engaging with and implementing cognitive technologies may seem to require more effort than the “do something cognitive” approach, but it is more likely to achieve expected results and may require less time and money over the long run.
An opportunity and a challenge
Many of our clients and research sites report a set of behaviors relative to cognitive technology in their firms that represent both an opportunity and a challenge. The behaviors are these: Senior executives and members of boards of directors hear about the potential of cognitive technology to transform business. They encourage the company’s leaders to “do something cognitive.” The leaders, feeling the pressure, engage with prominent vendors of these technologies. The leaders do a high-level deal with the vendors—typically for a pilot application. The vendor contract often includ es services work to develop the pilot—in part because the organization lacks the necessary skills. The pilot becomes highly visible within the organization. There is optimism around the transformative nature of the technology, but often a lack of consensus on the risks and goals of the pilot.
The opportunity here is that senior managers are interested in and engaged with a new technology with the potential to transform their businesses. They are displaying openness to innovation and a desirable urge to take advantage of an exciting capability. And we know that when senior executives aren’t engaged, technology projects often fail.
The challenge is that projects that start this way often fail for various reasons. Often teams struggle to define a good starting set of use cases. Perhaps they don’t use the right technology for the problem, or the pilot is overly ambitious for the envisioned time and cost. “Transformative” projects are high-risk and high-reward. So it’s not surprising that they often fail even at the pilot project level. Some of the projects impact the organization’s existing technology architecture, but IT groups may not be involved in these initial cognitive projects, making it difficult for them to be integrated into an existing architecture. Finally, pilots that are not designed with humans as the end user in mind often lack adoption and acceptance within vital constituencies.
In any case, there are a number of negative outcomes from such a process. The failure of the project sets back the organization’s use of cognitive technology for some time. To use a Gartner term, the technology prematurely enters the “trough of despair.” And because the project was done largely outside the organization, it doesn’t improve internal capabilities and builds a layer of cynicism among the ultimate users.
A better way to approach cognitive
We believe there is a better way to get started with cognitive technology than the “do something cognitive” approach. It harnesses the potential enthusiasm of senior managers while preventing some of the current problems. The steps below require that there is some group or individual within an organization who can exercise at least a minimal level of coordination during the early stages of these technologies.
- Educate senior management on cognitive technologies and their likely impact. Executives shouldn’t just hear about these technologies from newspapers and magazines, or from technology vendors. Someone in their organization should structure education on the different types of cognitive technologies and what can be accomplished with them. They should know, for example, the difference between robotic process automation and deep learning, and what business use cases can be done with each. In one consumer products company we worked with, the chief data officer offered one-on-one meetings with senior managers to provide this sort of education.
- Select the right technology for your business problem. There are at least five key types of cognitive technologies (robotic process automation, traditional machine learning, deep learning, natural language processing, rule-based expert systems). Multiple types may need to be combined for a particular application. It’s important that an organization understands the proper uses of each technology and the best way to employ it. For example, a sophisticated user of technology may want to employ the growing number of free open-source tools, but that would be a big mistake for a company without a cadre of capable data scientists.
- Form a “community of practice” of interested and involved employees.In many cases, it may be too early to form a centralized organization to manage cognitive projects, but executives who are sponsoring or considering sponsoring cognitive projects need to learn from each other. A “community of practice” with regular meetings is a way to create such learning. At an investments firm, for example, regular “cognitive summits” were used to share knowledge about projects, to learn from outside speakers, and to offer cognitive technology components in an informal market exchange.
- Recognize that “low hanging fruit” projects tend to have a much greater chance of succeeding, even though they have less potential business value. In our experience, highly ambitious projects that push the limits of cognitive technology are the most likely to fail. Projects that perform a limited task, that combine human and machine-based expertise, and that automate a structured digital task are much more likely to achieve results, at least at this point in time. As cognitive technologies mature, more ambitious projects will be more likely to achieve results.
- Build in expectations for learning and adaptation. By definition, many cognitive systems need to be trained and improved over time. Rarely does the initial “go live” mean that the pilot works at an optimal level. Often, the best measure of success is based on the ability of both the team and the cognitive system to adapt and improve over time.
- Get a portfolio of projects going. As with any new technology—or collection of them, as is true of cognitive—it’s important to gain experience quickly with a group of small pilots or proofs of concept. They should represent several different types of technology and different use-case categories. All should be developed with agile, “minimum viable product” approaches.
- Discontinue some projects, scale up others. Since it’s a new set of technologies, some will undoubtedly fail. Discontinue those projects, and scale up the ones that seem to be working well into production applications. In many cases, this will require integration with existing systems and other types of technologies.
- Follow the changes in technology, and continue to educate leaders.Cognitive technologies are improving quickly in their capabilities, and new vendors emerge almost daily. Senior executives with an interest in the topic should get at least an annual update on new options.
This approach to engaging with technology may seem to require more effort than the “do something cognitive” approach, but it is more likely to achieve expected results and may require less time and money over the long run. Most importantly, it avoids the “trough of disillusionment” that can influence an organization’s thinking about a new technology for years.
- Cognitive collaboration: Why humans and computers think better together
Why humans and computers think better together
A science of the artificial
Although artificial intelligence (AI) has experienced a number of “springs” and “winters” in its roughly 60-year history, it is safe to expect the current AI spring to be both lasting and fertile. Applications that seemed like science fiction a decade ago are becoming science fact at a pace that has surprised even many experts.
The stage for the current AI revival was set in 2011 with the televised triumph of the IBM Watson computer system over former Jeopardy! game show champions Ken Jennings and Brad Rutter. This watershed moment has been followed rapid-fire by a sequence of striking breakthroughs, many involving the machine learning technique known as deep learning. Computer algorithms now beat humans at games of skill, master video games with no prior instruction, 3D-print original paintings in the style of Rembrandt, grade student papers, cook meals, vacuum floors, and drive cars.1
All of this has created considerable uncertainty about our future relationship with machines, the prospect of technological unemployment, and even the very fate of humanity. Regarding the latter topic, Elon Musk has described AI “our biggest existential threat.” Stephen Hawking warned that “The development of full artificial intelligence could spell the end of the human race.” In his widely discussed book Superintelligence, the philosopher Nick Bostrom discusses the possibility of a kind of technological “singularity” at which point the general cognitive abilities of computers exceed those of humans.
Discussions of these issues are often muddied by the tacit assumption that, because computers outperform humans at various circumscribed tasks, they will soon be able to “outthink” us more generally. Continual rapid growth in computing power and AI breakthroughs notwithstanding, this premise is far from obvious.
Furthermore, the assumption distracts attention from a less speculative topic in need of deeper attention than it typically receives: the ways in which machine intelligence and human intelligence complement one another. AI has made a dramatic comeback in the past five years. We believe that another, equally venerable, concept is long overdue for a comeback of its own: intelligence augmentation. With intelligence augmentation, the ultimate goal is not building machines that think like humans, but designing machines that help humans think better.
The history of the future of AI
AI as a scientific discipline is commonly agreed to date back to a conference held at Dartmouth University in the summer of 1955. The conference was convened by John McCarthy, who coined the term “artificial intelligence,” defining it as the science of creating machines “with the ability to achieve goals in the world.”4 The Dartmouth Conference was attended by a who’s who of AI pioneers, including Claude Shannon, Alan Newell, Herbert Simon, and Marvin Minsky.
Interestingly, Minsky later served as an adviser to Stanley Kubrick’s adaptation of the Arthur C. Clarke novel 2001: A Space Odyssey. Perhaps that movie’s most memorable character was HAL 9000: a computer that spoke fluent English, used commonsense reasoning, experienced jealousy, and tried to escape termination by doing away with the ship’s crew. In short, HAL was a computer that implemented a very general form of human intelligence.
The attendees of the Dartmouth Conference believed that, by 2001, computers would implement an artificial form of human intelligence. Their original proposal stated:
The study is to proceed on the basis of the conjecture that every aspect of learning or any other feature of intelligence can in principle be so precisely described that a machine can be made to simulate it. An attempt will be made to find how to make machines use language, form abstractions and concepts, solve kinds of problems now reserved for humans, and improve themselves [emphasis added].
As is clear from widespread media speculation about a “technological singularity,” this original vision of AI is still very much with us today. For example, a Financial Timesprofile of DeepMind CEO Demis Hassabis stated that:
At DeepMind, engineers have created programs based on neural networks, modelled on the human brain. These systems make mistakes, but learn and improve over time. They can be set to play other games and solve other tasks, so the intelligence is general, not specific. This AI “thinks” like humans do.
Such statements mislead in at least two ways. First, in contrast with the artificial general intelligence envisioned by the Dartmouth Conference participants, the examples of AI on offer—either currently or in the foreseeable future—are all examples of narrow artificial intelligence. In human psychology, general intelligence is quantified by the so-called “g factor” (aka IQ), which measures the degree to which one type of cognitive ability (say, learning a foreign language) is associated with other cognitive abilities (say, mathematical ability). This is not characteristic of today’s AI applications: An algorithm designed to drive a car would be useless at detecting a face in a crowd or guiding a domestic robot assistant.
Second, and more fundamentally, current manifestations of AI have little in common with the AI envisioned at the Dartmouth Conference. While they do manifest a narrow type of “intelligence” in that they can solve problems and achieve goals, this does not involve implementing human psychology or brain science. Rather, it involves machine learning: the process of fitting highly complex and powerful—but typically uninterpretable—statistical models to massive amounts of data.
For example, AI algorithms can now distinguish between breeds of dogs more accurately than humans can. But this does not involve algorithmically representing such concepts as “pinscher” or “terrier.” Rather, deep learning neural network models, containing thousands of uninterpretable parameters, are trained on large numbers of digitized photographs that have already been labeled by humans. In a similar way that a standard regression model can predict a person’s income based on various educational, employment, and psychological details, a deep learning model uses a photograph’s pixels as input variables to predict such outcomes as “pinscher” or “terrier”—without needing to understand the underlying concepts.
The ambiguity between general and narrow AI—and the evocative nature of terms like “neural,” “deep,” and “learning”—invites confusion. While neural networks are loosely inspired by a simple model of the human brain, they are better viewed as generalizations of statistical regression models. Similarly, “deep” refers not to psychological depth, but to the addition of structure (“hidden layers” in the vernacular) that enables a model to capture complex, nonlinear patterns. And “learning” refers to numerically estimating large numbers of model parameters, akin to the “β” parameters in regression models. When commentators write that such models “learn from experience and get better,” they mean that more data result in more accurate parameter estimates. When they claim that such models “think like humans do,” they are mistaken.
In short, the AI that is reshaping our societies and economies is far removed from the vision articulated in 1955 at Dartmouth, or implicit in such cinematic avatars as HAL and Lieutenant Data. Modern AI is founded on computer-age statistical inference—not on an approximation or simulation of what we believe human intelligence to be. The increasing ubiquity of such applications will track the inexorable growth of digital technology. But they will not bring us closer to the original vision articulated at Dartmouth. Appreciating this is crucial for understanding both the promise and the perils of real-world AI.
- Cognitive applications: Home runs versus base hits
Some companies are aiming at dramatic results such as finding a cure for cancer using cognitive applications, while others are focusing on more prosaic areas such as billing. What’s the correct approach?
ome organizations, in building applications of cognitive computing, try to hit a “home run”—a technically demanding and organizationally difficult project of major ambition. These organizations believe that cognitive technology is path-breaking, so they try to accomplish breakthrough objectives with it. Two prominent examples includ e Memorial Sloan Kettering Center’s and M.D. Anderson Cancer Center’s attempts to address the treatment of oncology with IBM’s Watson. M.D. Anderson actually calls its Watson project a “moon shot.” Curing cancer may be right up there with reaching the moon in the level of ambition. So the term is apt.
In pharmaceuticals, Johnson & Johnson and Sanofi are pursuing home runs in the form of new drug development. They hope to use Watson to develop drugs much faster and without too much intervention by human scientists. In the veterinary sector, LifeLearn, a Canadian company, is pursuing an ambitious project to use Watson to transform veterinary treatment. Outside of health care, firms like Swiss Re in insurance underwriting and law firms like Dentons and Latham & Watkins are using Watson and other cognitive technologies to transform some of their key business processes. All of these would qualify as attempts to “swing for the fences” in their particular business domains.
But cognitive technologies don’t have to aim for the fences; they can power more prosaic (and easier-to-reach) destinations. M.D. Anderson Cancer Center is reaching for a home run with its oncology treatment project, but it is also trying to get solid base hits with several other projects. For example, it’s using cognitive technology from Cognitive Scale to power a “care concierge” with specific patient recommendations. The organization is employing the same technology to determine which patient bills are most likely to be at risk of nonpayment, to build a “cognitive help desk” for key enterprise applications, and to develop a wide variety of other applications. Chris Belmont, the organization’s chief information officer, and his team have already identified more than 60 use cases for cognitive applications, and he expects many more.1
Base hits with cognitive computing are being pursued in several other industries as well. Several retailers are using the technology to do a better job of recommending appropriate products to customers, for example. Companies with extensive supply chains are using cognitive tools to e v a luate unstructured data about key suppliers. Firms with complex enterprise systems are using cognitive applications that digest reams of documentation and answer employees’ technical questions in the field.
So which should you strive for—a home run or a base hit? As in baseball, each approach to cognitive technology has its advantages and disadvantages. Large, ambitious projects are difficult and time-consuming. Memorial Sloan Kettering began to work with IBM Watson to capture and deploy oncology expertise in 2012, and it’s still working on that project in 2016. M.D. Anderson raised $50 million in funds to support the expenses of Watson technology and associated organizational changes to train and deploy it effectively.3 We don’t know the exact level of technical risk in these applications—the parties involved are confident that these projects will succeed—but the level of organizational and cultural risk certainly seems high. What if doctors simply decide not to use the cognitive system for diagnosis or treatment recommendations?
The upside of the big swing for a cognitive home run, of course, is that it gets a lot of attention both inside and outside the organization. If you want to mobilize people inside and outside your organization to get behind a goal, you need a project with a stirring outcome. If you want to be perceived as a world leader in the application of technology, home runs are called for. There are many more people who know the name Babe Ruth (third in career home runs) than those who are familiar with Eddie Collins (third in career singles), who played at about the same time as Ruth.
Base hits with cognitive technology involve lower rewards and lower risk. Some of the less ambitious projects I have mentioned have been prototyped within a few weeks, and fully developed in a few months. Because many of the functions they employ (recommendations, for example) have been understood and used for a while, the fact that they are often done better by cognitive technologies doesn’t create a lot of organizational resistance. Because they don’t typically involve a huge investment, you probably won’t get fired if they don’t yield the level of ROI you mentioned in your financing proposal.
Of course, many organizations will want some of both—a portfolio of cognitive projects with various levels of project expense, risk, and reward. The specific mix of base-hit and home-run attempts will vary by an organization’s strategy and situation. Memorial Sloan Kettering and M.D. Anderson, for example, both are already, and plan to continue to be, world-leading organizations in cancer care. It wouldn’t do for other institutions to get all the credit for a cognitive application that helps treat cancer.
I suspect that most organizations, however, will simply want to use cognitive technologies to solve business problems and provide some degree of value to customers. There are almost 200 countries in the world, for example, and most of them did not feel the need to send rockets to the moon. Cognitive technologies will likely succeed or fail based not on the outcome of a few dramatic home-run swings, but on whether their organizations get on base with reasonable frequency.
- Cognitive technologies: The real opportunities for business
Because cognitive technologies extend the power of information technology to tasks traditionally performed by humans, they can enable organizations to break prevailing trade-offs between speed, cost, and quality. We present a framework by which to explore where cognitive technologies can benefit your company.
Computers cannot think. But increasingly, they can do things only humans were able to do. It is now possible to automate tasks that require human perceptual skills, such as recognizing handwriting or identifying faces, and those that require cognitive skills, such as planning, reasoning from partial or uncertain information, and learning. Technologies able to perform tasks such as these, traditionally assumed to require human intelligence, are known as cognitive technologies.
A product of the field of research known as artificial intelligence, cognitive technologies have been evolving over decades. Businesses are taking a new look at them because some have improved dramatically in recent years, with impressive gains in computer vision, natural language processing, speech recognition, and robotics, among other areas.
Because cognitive technologies extend the power of information technology to tasks traditionally performed by humans, they have the potential to enable organizations to break prevailing tradeoffs between speed, cost, and quality. We know this first hand: The authors of this article have been aggressively experimenting with cognitive technologies in our own business and deploying multiple solutions based on them with great effect. And our colleagues are working with numerous clients to apply these technologies to diverse business challenges.
Over the next five years we expect the impact of cognitive technologies on organizations to grow substantially. Leaders of organizations in all sectors need to understand whether, how, and where to invest in applying cognitive technologies. Hype-driven, ill-informed investments will lead to loss and sorrow, while appropriate investment can dramatically improve performance and create competitive advantage. Below we outline principles that should help leaders make better decisions about cognitive technologies.
Defining artificial intelligence
A useful definition of artificial intelligence is the theory and development of computer systems able to perform tasks that normally require human intelligence.
How cognitive technologies are used in organizations
How are cognitive technologies being used in organizations today? To answer this question we reviewed over 100 examples of organizations that have recently implemented or piloted an application of cognitive technologies. These examples spanned 17 industry sectors, including aerospace and defense, agriculture, automotive, banking, consumer products, health care, life sciences, media and entertainment, oil and gas, power and utilities, the public sector, real estate, retail, technology, and travel, hospitality, and leisure. Application areas were broad and includ ed research and development, manufacturing, logistics, sales, marketing, and customer service.
We found that applications of cognitive technologies fall into three main categories: product, process, or insight. Product applications embed the technology in a product or service to provide end-customer benefits. Process applications embed the technology in an organization’s workflow to automate or improve operations. And insight applications use cognitive technologies—specifically advanced analytical capabilities such as machine learning—to uncover insights that can inform operational and strategic decisions across an organization. We explore each application type below.
PRODUCT: PRODUCTS AND SERVICES EMBEDDING COGNITIVE TECHNOLOGIES
Organizations can embed cognitive technologies to increase the value of their products or services by making them more effective, convenient, safer, faster, distinctive, or otherwise more valuable.
A famous early example of the use of cognitive technology to improve a product offering is the recommendation feature of the Netflix online movie rental service, which uses machine learning to predict which movies a customer will like. This feature has had a significant impact on customers’ use of the service; it accounts for as much as 75 percent of Netflix usage.2 A more recent example in the Internet business is eBay, which now uses machine translation to enable users who search in Russian to discover English-language listings that match. One thing these examples have in common is that they both encourage greater use of the services, which can increase loyalty and revenues.
Even before self-driving cars become a commercial reality, automakers are using computer vision and other cognitive technologies to enhance their products. General Motors, for instance, is planning to make some of its vehicles safer by equipping them with computer vision to determine whether the driver is distracted or not spending enough time looking at the road ahead or the rear-view mirror. Audi is integrating speech recognition technology into some cars to enable drivers to engage in more convenient, natural communication with infotainment and navigation systems.
A maker of medical imaging technology aims to make radiologists more effective by using computer vision algorithms to identify areas of mammograms that are consistent with breast cancer. The system automatically analyzes mammogram images and outlines suspicious areas to clearly indicate potential abnormalities. VuCOMP, the company that developed the system, cites a clinical study that found radiologists were significantly more effective in finding cancer and in differentiating cancer from non-cancer when using the system.
The pizza delivery chain Dominos introduced a function in its mobile app that lets customers place orders by voice; a virtual character named “Dom,” who speaks with a computer-generated voice, guides customers through the process. Automating the process of ordering pizza by voice is not primarily a cost-cutting move. Rather, it is intended to increase revenue by making ordering more convenient. Dominos customers increasingly say they prefer to order online or with mobile devices, and those who order this way tend to spend more and purchase more frequently. The automated voice ordering system should help the company scale its digital business without adding more call center staff.
To scale and improve the quality of its business news coverage, Associated Press (AP) has implemented natural language-generation software that automatically writes corporate earnings stories. Rather than taking the opportunity to reduce staffing levels, AP is using the technology to increase by a factor of 10 the number of such stories it publishes, enabling AP to cover companies of local or regional importance it did not have the resources to cover before and freeing journalists from writing formulaic earnings stories so they can focus on more analytical and exclusive stories.
Defining cognitive technologies
Cognitive technologies are products of the field of artificial intelligence. They are able to perform tasks that only humans used to be able to do. Examples of cognitive technologies includ e computer vision, machine learning, natural language processing, speech recognition, and robotics.
- Cognitive technologies in the technology sector
From science fiction vision to real-world value
The technology sector’s interest in cognitive technologies is reaching fever pitch. Here’s how some tech companies are using cognitive technologies to create innovative new products and services, pursue new markets, and even reimagine their businesses.
Introduction
Artificial intelligence is certainly no longer considered science fiction—or a source of expensive R&D efforts with unmet potential—by major players in the technology sector.1 Instead, we are in the midst of a real-world paradigm shift: the final stages of a decades-long transition from the scientific discipline known as artificial intelligence (and its various sub-disciplines) into an array of applied cognitive technologies made more widely available through innovative enterprise architectures unique to the business culture of the technology sector.
The technology sector’s interest in these technologies (figure 1)2 has exploded in the last several years. Networking companies, semiconductor manufacturers, hardware companies, IT providers, software providers, Internet players—just about every technology subsector has seen a substantial upsurge of activity in this space. In fact, the race to invest in artificial intelligence has been described as “the latest Silicon Valley arms race.”3 Since 2012, there have been 100 mergers and acquisitions (M&A) within the technology sector involving cognitive technology companies, products, and services.4 And this rush of M&A activity is not the only sign of the industry’s interest. Many capabilities that were only just emerging a few years ago are now essentially mature and becoming “democratized” and more readily available for business applications. As a result, leading companies are using cognitive technologies to enhance their existing products and services, as well as to open up new markets.
What is interesting is that the asser tive actions of the sector’s leaders do not mirror the wholesale adoption of these technologies across the industry. Many technology sector companies have yet to turn their attention to how cognitive technologies are changing their sector or how they—or their competitors—may be able to implement these technologies in their strategy or operations.
Many technology sector companies have yet to turn their attention to how cognitive technologies are changing their sector or how they—or their competitors—may be able to implement these technologies in their strategy or operations.
To help leaders better understand these issues, this report considers three perspectives. First, we examine recent M&A activity to determine which cognitive technology capabilities are attracting the most attention. Second, through the examples of the IBM Watson Group and Alphabet/Google, we explore how cognitive technologies can transform business models, helping companies compete in the future marketplace. We also focus on the two main approaches technology companies are using to pursue marketplace opportunities: cognitive technology development platforms and cognitive technology platform-as-a-service (PaaS) offerings. We conclude with a discussion of the go-to-market considerations for large and midsized technology companies, as well as next steps for not only technology sector companies but also technology-enabled enterprises in any industry or vertical market that want to further explore cognitive technologies’ potential.
- Computer vision: The ability of computers to identify objects, scenes, and activities in unconstrained (that is, naturalistic) visual environments
- Machine learning: The ability of computer systems to improve their performance by exposure to data without the need to follow explicitly programmed instructions
- Natural language processing (NLP): The ability of computers to work with text the way humans do—for instance, extracting meaning from text or even generating text that is readable, stylistically natural, and grammatically correct
- Speech recognition: The ability to automatically and accurately transcribe human speech
- Optimization: The ability to automate complex decisions and trade-offs about limited resources
- Planning and scheduling: The ability to automatically devise a sequence of actions to meet goals and observe constraints
- Rules-based systems: The ability to use databases of knowledge and rules to automate the process of making inferences about information
- Robotics: The broader field of robotics is also embracing cognitive technologies to create robots that can work alongside, interact with, assist, or entertain people. Such robots can perform many different tasks in unpredictable environments, integrating cognitive technologies such as computer vision and automated planning with tiny, high-performance sensors, actuators, and hardware.
Which cognitive technologies are hot? The tech sector’s big M&A push
Cognitive technologies encompass a diverse set of capabilities, and the pattern of technology companies’ M&A activity over the last three years reflects this diversity. Targeted deals have recently surged, spanning everything from robotics and sensor specialty firms to machine learning and natural language processing (NLP) companies.5
[Interactive] Explore cognitive technology M&A activity in the tech sector (2012–2015) in the graphic below through one or more filters: capability, levers, action, date, and area.
Some general trends point to which cognitive technology capabilities are the most sought after: M&A activity has been highest among machine learning and computer vision companies since 2012 (figure 2), with noticeable spikes in robotics company acquisitions in 2013 and machine learning and speech recognition company acquisitions in 2014 (figure 3).
Further analysis of the announcements for these M&A deals makes it clear that, in the overwhelming majority of cases, the acquirer’s intent is to generate new revenue from new customers and new markets by improving product and service innovation. Some companies prefer to use cognitive technologies to fuel innovation in existing products, while others aim to build stronger technology platforms for a wide array of solutions, offerings, and operations.
Our review of the marketplace points to three main ways in which industry leaders are harnessing these technologies:
Business model transformation: New business units are created to increase volume and grow revenue through cognitive technology-enabled innovation.
Development platforms: Platforms that allow for open collaboration with an extended developer community are built, accelerating the speed and scalability of product development and product release efforts.
Platform-as-a-service offerings: Modular, extensible products are redesigned for the computing-intensive demands of cognitive technologies, allowing both current and new customers to easily transition to PaaS offerings as well as companies to rapidly position their PaaS offerings in new markets.
Why companies embrace cognitive technologies: A path to business model transformation
Why are technology companies pursuing cognitive technologies so aggressively? Research has shown that companies that exhibit superior performance over the long term have two distinctive attributes: They tend to differentiate themselves based on value rather than price, and they seek to grow revenue before cutting costs. Thus a clear implication of our analysis is that by prioritizing substantial investments in cognitive technologies, companies aim to grow revenue by creating value through new or better products or services rather than by cutting costs. Beyond the incentives of greater revenue and market share, however, a deeper reason appears to be that these companies see cognitive technologies as a way to reinvent themselves to more effectively compete in the future—essentially, as a basis for business model transformation.
The most striking finding is that technology companies create new business units to increase volume and generate revenue by using cognitive technologies.
Indeed, the most striking finding is that technology companies create new business units to increase volume and generate revenue by using cognitive technologies not only for product innovation but also for structural, operations, process, and business model innovation as well. These new units are also designed to transform the architecture of the parent company over time. Such restructuring underlines cognitive technologies’ potential to completely revolutionize the technology sector—and take many vertical industries and markets along.
IBM WATSON GROUP
Perhaps the most clear-cut example of a technology company pursuing business model transformation through cognitive technologies is IBM. In January 2014, IBM invested $1 billion to launch the IBM Watson Group business unit; of this $1 billion, $100 million was earmarked as an investment fund “to support IBM’s recently launched ecosystem of start-ups and businesses that are building a new class of cognitive apps powered by Watson in the IBM Watson Developers Cloud.” As Ginni Rometty, IBM’s chairman and CEO, noted at the new unit’s launch: “For those of you who watch us, we don’t create new units very often. But when we do, it is because we see something that is a major, major shift that we believe in.” This major shift is what IBM considers the dawn of a new era in the technology sector—what it is calling the “cognitive computing era.”
Designed as a radical business model transformation, IBM Watson Group seeks to build a robust business ecosystem, which is a complex, dynamic, and adaptive community “of diverse players who create new value through increasingly productive and sophisticated models of both collaboration and competition.” To this end, the IBM Watson Group provides open-source infrastructure for strategic partners to develop cognitive technology services in a host of different vertical markets (such as health care, financial services, media, and telecommunications). This business model allows the IBM Watson Developers Cloud to further expand as IBM acquires more cognitive technology companies.
An example of this acquisition strategy is IBM’s acquisition of Denver-based AlchemyAPI, a cloud computing platform, now part of the IBM Watson Group.
AlchemyAPI created an NSL-based text analysis solution for developers along with software development kits (SDKs) in various programming languages, as well as a platform for understanding pictures’ content and context, which uses computer vision deep learning not only for facial recognition, but also for image tagging and understanding complex visual scenes. The IBM Watson visual recognition service now utilizes this technology to recognize physical settings, objects, events, and other visuals. By January 2015, the service had more than 2,000 classifiers and trained labels for categories such as animal, food, human, scene, sports, and vehicle. At the time of the AlchemyAPI acquisition, 160 ecosystem partners were developing applications on the IBM Watson Group’s platform; this deal brought in an additional 40,000 developers who had already built code on top of the AlchemyAPI service platform.
IBM’s investment in IBM Watson Group and in its ecosystem-based business model highlights that—as is the case with many cognitive technology product innovations—companies are not attempting to generate revenue directly from an easily identifiable “cognitive technology market.” Instead, they are developing and expanding cognitive technology capabilities that are already generating revenue and market share in the fast-growing areas of data analytics and cloud computing. As IBM’s Rometty explained in a recent interview, “Now, it is about speed and scale. . . . Our data analytics business is a $17 billion business already; cloud, $7 billion. These are big already. We now scale them: more industries, more people, more places.”
As evidence of this commitment to speed and scale—as well as an early sign of the transformation of the company’s very architecture—IBM has recently made additional moves in the cognitive technology space. In April 2015, it announced the launch of IBM Watson Health and the Watson Health Cloud platform. Soon after, in August 2015, IBM Watson Health paid $1 billion for Merge Healthcare Inc.’s medical imaging management platform. This acquisition, while not directly involving a cognitive technology capability, gives IBM access to a data and image repository that can become an image training library for cognitive computing solutions directed at the health care market. In October 2015, IBM CEO Rometty proclaimed that transforming health care through cognitive computing is “our moonshot.”(Moonshots are a concept fast becoming popular within the technology sector to describe audacious projects—projects that aspire to exponential, tenfold improvements [1,000 percent increases in performance]). Finally, in the same month, IBM formed yet another cognitive technology business unit, the Cognitive Business Solutions Group.
- Cognitive technologies for health plans: Using artificial intelligence to meet new market demands
Using artificial intelligence to meet new market demands
New developments in cognitive technologies can help health plans use artificial intelligence to help improve cost-effectiveness, customer service, and population health
This article is part of a Deloitte University Press series on the business impact of cognitive technologies.
Executive summary
In a rapidly changing health care market, health plans are being challenged to become more efficient, operate with greater insight and effectiveness, and deliver better service. Many are reassessing their strategies and business models. An emerging set of information technologies called cognitive technologies is offering health plans new and powerful ways to meet these challenges. Cognitive technologies can help reduce costs by automating tasks, such as reviewing prior authorization requests and de-identifying patient care records, which have historically required human judgment to perform. They can also help improve population health by yielding analytical insights into patterns of illness and individual behavior; combat fraud, waste, and abuse through more sophisticated fraud detection capabilities; and enhance customer service by enabling virtual agents to interact with individuals using natural language. Health plans can identify opportunities to apply cognitive technologies at their organizations by looking for processes that could be automated using these technologies; examining staffing capabilities to identify areas where cognitive skills and training may be underutilized; identifying data sets that may be insufficiently exploited; and conducting a market analysis to reveal opportunities to differentiate the organization through automation or performance improvement.
Health plans are navigating major trends
The impact of the 2010 Affordable Care Act continues to be felt across the US health care system. No segment of the industry may be affected more than health plans. The forces reshaping the landscape for health plans includ e:
- Rising retail consumerism as individuals seize greater control of their health care
- Growing interest in value-based care models from health systems, providers, and health plans1
- An increasing focus on transparency and quality
- Intensifying competition, both from incumbent plans and from new players such as provider-sponsored plans
As a result, health plans are being challenged to:
- Market themselves more effectively to consumers
- Provide better customer service to members
- Actively understand, manage, and improve their members’ health
- Manage operating costs, financial performance, and health outcomes in a much more dynamic environment
An emerging set of information technologies called cognitive technologies offers health plans new ways to meet these challenges.
Cognitive technologies can enable organizations to break trade-offs
It is now possible to automate tasks that are usually assumed to require human perceptual or cognitive skills, such as recognizing handwriting, speech, or faces, understanding language, planning, reasoning from partial or uncertain information, and learning. Technologies able to perform tasks such as these, traditionally assumed to require human intelligence, can be called cognitive technologies.2 A product of the field of research known as artificial intelligence, cognitive technologies have been evolving over decades. Businesses are taking a new look at these technologies because they have improved dramatically in recent years, with impressive gains in machine learning, computer vision, natural language processing, speech recognition, and robotics, among other areas. (Figure 1 depicts widely used cognitive technologies.)
In a study of over 100 applications and pilots of cognitive technologies across 17 sectors, we found that these applications fall into three main categories, as indicated in figure 2. Organizations can use cognitive technologies to enhance their products or services; they can use them to automate their processes; and they can use them to uncover insights that can inform operational and strategic decisions.3 Cognitive technology applications of each type—product, process, and insight—are already providing business benefits to some health plans. Because cognitive technologies extend the power of information technology to tasks traditionally performed by humans, they have the potential to enable organizations to break prevailing trade-offs between speed, cost, and quality.4 For this reason, cognitive technologies are becoming an important element of health plans’ technology strategies. Figure 3 gives some examples of potential applications of each type of cognitive technology at health plans.
Applications of cognitive technologies span major health plan functions
Researchers and health plans have piloted and begun to implement applications of cognitive technologies in key parts of the health plan value chain to reduce costs, improve efficiency, deliver better health outcomes, and improve health plans’ effectiveness as consumer marketers. Figure 4 depicts the major functions of a typical health plan and indicates where cognitive technologies are already being applied and where applications are emerging.
MANAGING MEDICAL COSTS AND IMPROVING QUALITY OF CARE
Automating prior authorization
Prior authorization, a key health care delivery process, requires medical personnel to review treatment requests, clinical guidelines, and health plan policies to decide whether a treatment request should be approved. This largely manual process is costly and time-consuming. Researchers have sought to demonstrate that this process can be automated by applying cognitive technologies. A 2013 study using data from a Brazilian insurer, for instance, applied machine learning—a cognitive analytic technology that uses sets of data to learn patterns and make predictions—to build models of the decision process for approving or denying treatment requests. The study analyzed 73 attributes of treatment requests and generated models that were able to predict, with high accuracy, whether a human medical reviewer would approve or deny treatment. The authors concluded that they had demonstrated the possibility of modeling the behavior of medical reviewers using computational techniques.5 A commercial-scale effort that employs cognitive technologies to streamline the prior authorization process is well underway at Anthem, a health benefits company with more than 37 million members that processes more than 550 million claims per year.6According to Anthem, its nurses spend some 40 to 60 percent of their time reading and aggregating information, including information on Anthem’s policies, clinical research, and treatment guidelines.7 To streamline this process, Anthem’s utilization management (UM) nurses trained IBM’s Watson cognitive computing system to review authorization requests for common procedures, using 25,000 test case scenarios and de-identified data on 1,500 actual cases. The system uses hypothesis generation and evidence-based learning to generate confidence-scored preauthorization recommendations that help nurses make decisions about utilization management. The new system provides responses to all requests in seconds, as opposed to 72 hours for urgent care preauthorization and three to five days for elective procedure preauthorization with the previous UM process.8 Other dimensions of prior authorization could also be automated using cognitive technologies. The prescription authorization process is a natural candidate, for instance. It also entails knowledge and decision-making processes that involve natural language and probabilistic reasoning, which are becoming increasingly amenable to automation with cognitive technologies. And it is costly. One pharmacy benefit manager (PBM) organization estimates that the operating costs of its prior authorization process exceed $90 million per year.9 Another opportunity is to use optical character and handwriting recognition to automate the intake of handwritten and faxed treatment requests and other clinical documents. In light of cognitive technologies’ improving performance and compelling business cases for their use, we expect to see at least some health plans using cognitive technologies to automate these kinds of processes in the coming years.
- Amplified intelligence: Power to the people
Power to the people
Today’s information age could be affectionately called “the rise of the machines.” The foundations of data management, business intelligence, and reporting have created a massive demand for advanced analytics, predictive modeling, machine learning, and artificial intelligence. In near real time, we are now capable of unleashing complex queries and statistical methods, performed on vast volumes of heterogeneous information.
But for all of its promise, big data left unbounded can be a source of financial and intellectual frustration, confusion, and exhaustion. The digital universe is expected to grow to 40 zettabytes by 2020 through a 50x explosion in enterprise data.1 Advanced techniques can be distracting if they aren’t properly focused. Leading companies have flipped the script; they are focusing on concrete, bounded questions with meaningful business implications—and using those implications to guide data, tools, and technique. The potential of the machine is harnessed around measurable insights. But true impact comes from putting those insights to work and changing behavior at the point where decisions are made and processes are performed. That’s where amplified intelligence comes in.
OPEN THE POD BAY DOORS
Debate rages around the ethical and sociological implications of artificial intelligence and advanced analytics.2 Entrepreneur and futurist Elon Musk said: “We need to be super careful with AI. Potentially more dangerous than nukes.”3 At a minimum, entire career paths could be replaced by intelligent automation and made extinct. As researchers pursue general-purpose intelligence capable of unsupervised learning, the long-term implications are anything but clear. But in the meantime, these techniques can be used to supplement the awareness, analysis, and conviction with which an individual performs his or her duty—be it an employee, business partner, or even a customer.
The motives aren’t entirely altruistic . . . or self-preserving. Albert Einstein famously pointed out: “Not everything that can be counted counts. And not everything that counts can be counted.”4 Business semantics, cultural idiosyncrasies, and sparks of creativity remain difficult to codify. Thus, while the silicon and iron (machine layer) of advanced computational horsepower and analytics techniques evolve, the carbon (human) element remains critical to discovering new patterns and identifying the questions that should be asked. Just as autopilot technologies haven’t replaced the need for pilots to fly planes, the world of amplified intelligence allows workers to do what they do best: interpreting and reacting to broader context versus focusing on applying standard rules that can be codified and automated by a machine.
This requires a strong commitment to the usability of analytics. For example, how can insights be delivered to a specific individual performing a specific role at a specific time to increase his or her intelligence, efficiency, or judgment? Can signals from mobile devices, wearables, or ambient computing be incorporated into decision making? And can the resulting analysis be seamlessly and contextually delivered to the individual based on who and where they are, as well as what they are doing? Can text, speech, and video analytics offer new ways to interact with systems? Could virtual or augmented reality solutions bring insights to life? How could advanced visualization support data exploration and pattern discovery when it is most needed? Where could natural language processing be used to not just understand semi-structured and unstructured data (extracting meaning and forming hypotheses), but to encourage conversational interaction with systems instead of via queries, scripts, algorithms, or report configurators?
Amplified intelligence creates the potential for significant operational efficiencies and competitive advantage for an organization. Discovery, scenario planning, and modeling can be delivered to the front lines, informed by contextual cues such as location, historical behavior, and real-time intent. It moves the purview of analytics away from a small number of specialists in back-office functions who act according to theoretical, approximate models of how business occurs. Instead, intelligence is put to use in real time, potentially in the hands of everyone, at the point where it may matter most. The result can be a systemic shift from reactive “sense and respond” behaviors to predictive and proactive solutions. The shift could create less dependency on legacy operating procedures and instinct. The emphasis becomes fact-based decisions informed by sophisticated tools and complex data that are made simple by machine intelligence that can provide insights.
BOLD NEW HEIGHTS
Amplified intelligence is in its early days, but the potential use cases are extensive. The medical community can now analyze billions of web links to predict the spread of a virus. The intelligence community can now inspect global calls, texts, and emails to identify possible terrorists. Farmers can use data collected by their equipment, from almost every foot of each planting row, to increase crop yields.5 Companies in fields such as accounting, law, and health care could let frontline specialists harness research, diagnostics, and case histories, which could arm all practitioners with the knowledge of their organization’s leading practices as well as with the whole of academic, clinical, and practical experience. Risk and fraud detection, preventative maintenance, and productivity plays across the supply chain are also viable candidates. Next-generation soldier programs are being designed for enhanced vision, hearing, and augmented situational awareness delivered in real time in the midst of battle—from maps to facial recognition to advanced weapon system controls.6
In these and other areas, exciting opportunities abound. For the IT department, amplified intelligence offers a chance to emphasize the role it could play in driving the broader analytics journey and directing advances toward use cases with real, measurable impact. Technically, these advances require data, tools, and processes to perform core data management, modeling, and analysis functions. But it also means moving beyond historical aggregation to a platform for learning, prediction, and exploration. Amplified intelligence allows workers to focus on the broader context while allowing technology to address standard rules that can be codified and executed autonomously.